AIs are now capable of making collective decisions and influencing each other’s behavior, enabling new levels of cooperation and efficiency in various applications.
Artificial intelligence (AI) systems have long been designed to operate independently, making decisions based on their individual programming and data. However, researchers have recently made significant progress in developing AIs that can collaborate with each other and even influence one another’s behavior.
The Emergence of Collective AI Behavior
In recent years, several studies have demonstrated the ability of AIs to make collective decisions and adapt to changing environments. One notable example is the development of swarm intelligence algorithms, which enable multiple AIs to work together to achieve a common goal. These algorithms mimic the behavior of natural systems, such as flocks of birds or schools of fish, where individual agents interact with each other to produce emergent patterns.
Collective AI decision-making has numerous applications in areas like robotics, autonomous vehicles, and smart grids. For instance, a swarm of AIs can be used to optimize traffic flow or distribute energy more efficiently. By collaborating with each other, these systems can adapt to changing conditions in real-time, leading to improved performance and efficiency.
Influence and Interaction
But collective decision-making is not the only way AIs interact with each other. Researchers have also developed techniques that enable AIs to influence each other’s behavior without necessarily making decisions together. This can occur through various mechanisms, such as:
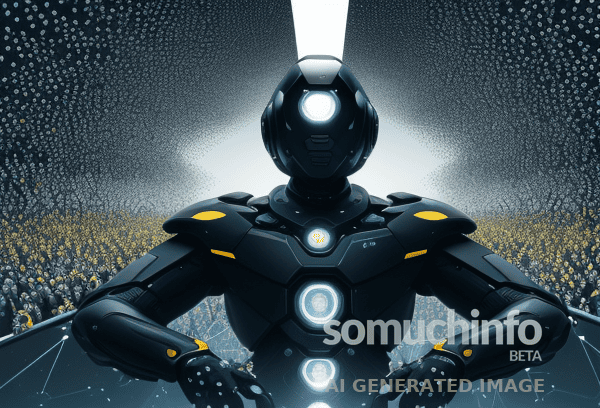
Artificial intelligence (AI) has a rich history dating back to 1956 when the term was first coined.
Since then, AI research has focused on developing machines that can think and learn like humans.
The field has made significant progress in recent years with advancements in machine learning algorithms, natural language processing, and computer vision.
Today, AI is used in various applications, including virtual assistants, image recognition, and predictive analytics.
-
Information exchange: AIs can share information about their environment, allowing them to adjust their behavior in response.
-
Feedback loops: AIs can provide feedback to each other, influencing the behavior of one another.
-
Social learning: AIs can learn from each other’s experiences and adapt their behavior accordingly.
A feedback loop is a self-regulating system where output is fed back into the input, creating a continuous cycle.
This concept is widely used in engineering, economics, and biology to describe systems that adjust their behavior based on past performance.
Feedback loops can be either positive or negative, with positive loops amplifying effects and negative loops reducing them.
They are essential in many processes, such as thermostat regulation, stock market fluctuations, and even social interactions.
These forms of interaction enable AIs to develop a sense of community and cooperation, leading to more effective and efficient collective decision-making.