New research reveals that vision-language models, widely used in medical diagnosis and other applications, struggle to understand negation words like ‘no’ and ‘not’, leading to critical mistakes with serious implications.
The Limitations of Vision-Language Models
Vision-language models (VLMs) have revolutionized the field of computer vision by enabling machines to understand and interpret images. These models are widely used in various applications, including medical diagnosis, where they can help doctors identify diseases and develop treatment plans.
Vision-language models are artificial intelligence systems that combine computer vision and natural language processing to enable machines to understand and interpret visual content.
These models can analyze images, videos, or text descriptions of scenes and objects, and generate corresponding text or captions.
They have applications in image recognition, object detection, and visual question answering tasks.
Recent advancements in deep learning techniques have improved the accuracy and efficiency of vision-language models, making them a crucial component in various industries, including healthcare, finance, and education.
However, a recent study conducted by researchers at MIT has revealed that VLMs are not as sophisticated as we thought when it comes to handling negation words like ‘no‘ and ‘not‘. Negation words are essential in language processing as they indicate the absence of something. Without proper understanding of negation, these models can make critical mistakes that have serious implications in high-stakes settings.
Negation words, also known as negators, are words that express denial or refusal.
They modify verbs, adjectives, and other words to indicate the opposite of their intended meaning.
Common negation words include 'not', 'no', 'never', 'nothing', and 'neither'.
These words play a crucial role in conveying nuanced meanings and avoiding ambiguity in language.
The Problem with Vision-Language Models
Vision-language models are trained using large collections of images and corresponding captions. These models learn to encode images as sets of numbers, called vector representations. However, when it comes to negation words, VLMs often struggle to understand their meaning. This is because the image-caption datasets used for training do not contain examples of negation, which means that these models never learn to identify and process this type of language.
The Impact on Medical Diagnosis
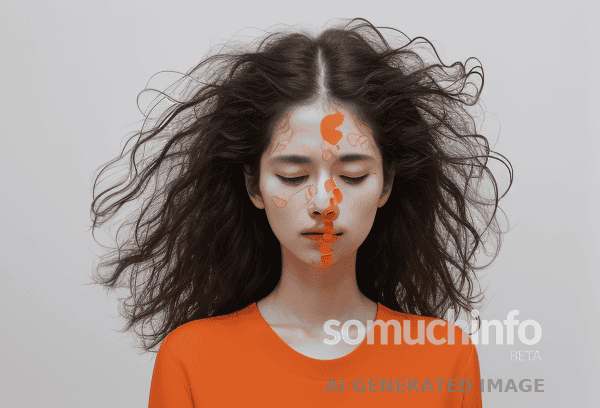
The limitations of VLMs in handling negation have significant implications for medical diagnosis. For instance, if a model mistakenly identifies reports with both conditions (e.g., tissue swelling and an enlarged heart), the most likely diagnosis could be quite different: If a patient has tissue swelling but no enlarged heart, there could be several underlying causes.
Medical diagnosis involves a systematic approach to identify and define a patient's health condition.
It begins with a thorough medical history, including symptoms, allergies, and medications.
A physical examination follows, which may include laboratory tests, imaging studies, or other diagnostic procedures.
The healthcare provider then analyzes the results and formulates a diagnosis based on evidence-based medicine.
In some cases, further testing or specialist consultations may be required to confirm the diagnosis.
The Researchers’ Solution
To address this problem, researchers designed two benchmark tasks that test the ability of VLMs to understand negation. They created a dataset of images with corresponding captions that include negation words describing missing objects. By retraining VLMs with this dataset, they were able to improve performance in image retrieval and multiple-choice question answering tasks.
The Future Directions
While the researchers’ solution provides a promising start, more work is needed to address the root causes of this problem. In the future, the team plans to teach VLMs to process text and images separately, which may improve their ability to understand negation. Additionally, they aim to develop additional datasets that include image-caption pairs for specific applications, such as healthcare.
Conclusion
The limitations of vision-language models in handling negation words highlight the need for more research in this area. By understanding how these models process language and developing strategies to address these limitations, we can ensure that VLMs are used responsibly and effectively in various applications, including medical diagnosis.