A groundbreaking technique, test-time augmentation, has been developed to improve the accuracy of artificial intelligence predictions in high-stakes settings, such as medical imaging, by reducing the size of prediction sets while maintaining confidence guarantees.
The ambiguity in medical imaging can present major challenges for clinicians who are trying to identify disease. For instance, in a chest X-ray, pleural effusion, an abnormal buildup of fluid in the lungs, can look very much like pulmonary infiltrates, which are accumulations of ‘pus or blood.’
Medical imaging is a diagnostic technique that uses various technologies to visualize the internal structures of the body.
It involves creating images of organs, tissues, and bones using different modalities such as X-rays, computed tomography (CT), magnetic resonance imaging (MRI), ultrasound, and positron emission tomography (PET).
Medical imaging helps doctors diagnose and monitor diseases, injuries, and conditions, allowing for timely and effective treatment.
According to the World Health Organization (WHO), medical imaging is a crucial tool in modern healthcare, accounting for over 70% of all diagnostic procedures worldwide.
One promising way to produce a set of possibilities, called conformal classification, is convenient because it can be readily implemented on top of an existing machine-learning model. However, it can produce sets that are impractically large. This approach has limitations, as the inherent uncertainty in AI predictions often causes the model to output sets that are far too large to be useful.
To overcome this challenge, researchers have developed a simple and effective improvement that can reduce the size of prediction sets by up to 30 percent while also making predictions more reliable. The technique is called test-time augmentation (TTA), which creates multiple augmentations of a single image in a dataset, applies a computer vision model to each version, and aggregates its predictions.
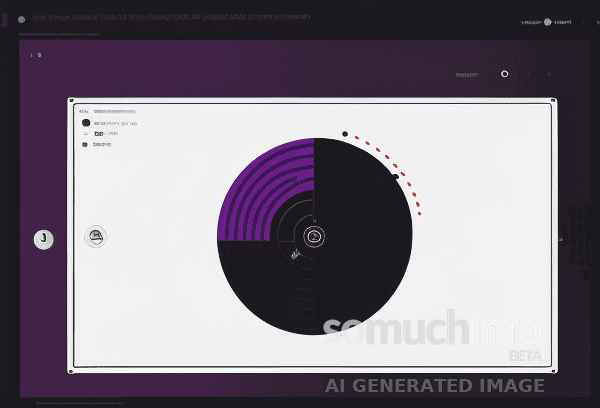
Test-time augmentation is a technique used to improve the performance of deep neural networks during inference.
It involves applying random augmentations, such as rotation or color jittering, to input images at test time.
This approach has been shown to enhance model robustness and accuracy on various tasks, including image classification and object detection.
By increasing the diversity of inputs, models learn to generalize better and become less susceptible to overfitting.
By applying TTA, the researchers found that the conformal classifier outputs a smaller set of probable predictions for the same confidence guarantee. This approach achieves this reduction in prediction set size while maintaining the probability guarantee. Moreover, the technique boosts accuracy enough to outweigh the cost of losing labeled data used for the conformal classification procedure.
A conformal classifier is a type of machine learning model that provides confidence scores for predictions.
These models are based on the idea of assigning a probability to each prediction, indicating how likely it is to be correct.
Conformal classifiers have been shown to improve the reliability and accuracy of predictions in various applications, including image classification and natural language processing.
They work by estimating the distribution of predictions using a set of reference data, allowing for more accurate confidence scoring.
The researchers also want to validate the effectiveness of such an approach in the context of models that classify text instead of images. To further improve the work, they are considering ways to reduce the amount of computation required for TTA. This research is funded, in part, by the Wistrom Corporation.