A groundbreaking new framework for machine learning discovery has been unveiled, revolutionizing the way researchers combine existing algorithms to create innovative AI models.
The field of machine learning has made tremendous progress in recent years, with various algorithms being developed to tackle complex problems. However, these ‘algorithms often operate in isolation, making it challenging for scientists to combine existing ideas and create new ones.’ Researchers at MIT have created a unifying framework that can help scientists fuse strategies from different methods to improve existing AI models or come up with new ones.
Machine learning is a subset of artificial intelligence that enables systems to learn from data without being explicitly programmed.
The first machine learning model was developed in the 1950s, but it wasn't until the 1990s that the field gained significant attention.
Since then, advancements in deep learning and neural networks have led to breakthroughs in image recognition, natural language processing, and predictive analytics.
The researchers developed a periodic table of machine learning that shows how more than 20 classical ‘algorithms are connected.’ This table categorizes each algorithm based on the approximate relationships it learns and provides a toolkit for designing new algorithms without rediscovering ideas from prior approaches.
The table is built around a unifying equation that underlies many classical AI algorithms, which learn a specific kind of relationship between data points. While each ‘algorithm may accomplish this in a slightly different way, the core mathematics behind each approach is the same.’ By reframing popular methods and arranging them into a table, the researchers identified gaps where algorithms could exist but have not been discovered yet.
The periodic table gives researchers a guide to design new algorithms without having to guess their way through. The flexible framework allows scientists to add new rows and columns to represent additional types of data point connections. By filling in these gaps, the researchers created a new algorithm that could classify unlabeled images 8 percent better than another state-of-the-art approach.
The creation of I-Con, an information contrastive learning framework, has opened up new avenues for discovery. This unifying approach provides a rich set of ‘algorithms spanning over 100 years of research in machine learning.’ By thinking outside the box and combining ideas in ways they wouldn’t necessarily have thought of otherwise, researchers can create more efficient and effective AI models.
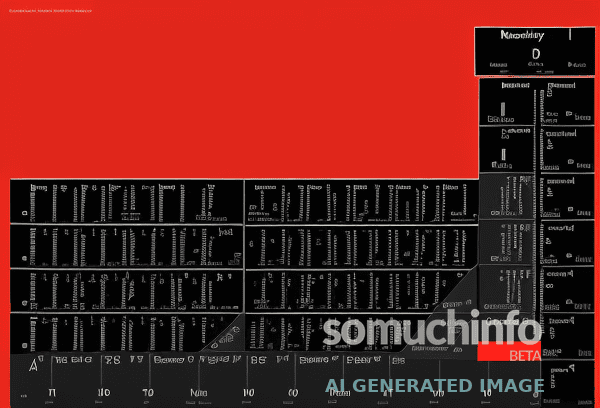
The development of I-Con is an excellent example of unifying and connecting existing algorithms. This research has the potential to inspire others to apply a similar approach to other domains of machine learning. With the increasing number of papers being published each year, it’s essential to have frameworks like I-Con that provide a clear understanding of how different algorithms can be combined.
-
Research
-
Computer science and technology
-
Artificial intelligence
-
Machine learning
-
Algorithms