As the world grapples with the rapid advancement of Artificial Intelligence (AI), many organizations are struggling to successfully develop and deploy AI products. A recent study reveals that only a small fraction of companies have achieved significant returns on their investments, highlighting the need for a systematic approach to AI transformation.
Only 26% of companies have successfully developed working AI products, and a mere 4% have achieved significant returns on their investments. This stark reality highlights the need for a systematic approach to AI transformation.
Artificial intelligence (AI) refers to the development of computer systems that can perform tasks that typically require human intelligence, such as visual perception, speech recognition, and decision-making.
AI has made significant progress in recent years, with applications in areas like healthcare, finance, and transportation.
According to a report by Gartner, the global AI market is expected to reach $190 billion by 2025.
Key players in the AI industry include Google, Microsoft, and Amazon, which are investing heavily in AI research and development.
Framework 1: The ‘The AI Risk Matrix’
To prime organizations for AI innovation, it is essential to consider the risk matrix. This involves evaluating the likelihood and potential impact of various risks associated with AI adoption. By doing so, companies can identify areas that require mitigation strategies and allocate resources accordingly.
The ‘AI risk matrix’ consists of four quadrants:
An AI risk matrix is a framework used to assess and categorize potential risks associated with 'artificial intelligence.' It evaluates the likelihood and impact of various AI-related threats, such as 'bias,' job displacement, or cybersecurity breaches. The matrix typically involves plotting risks on two axes: likelihood and impact. This allows organizations to prioritize and mitigate high-risk areas, ensuring responsible AI development and deployment.
-
High-Low: These are risks with a high likelihood but low impact.
-
Low-High: These are risks with a low likelihood but high impact.
-
High-High: These are risks with a high likelihood and high impact.
-
Low-Low: These are risks with a low likelihood and low impact.
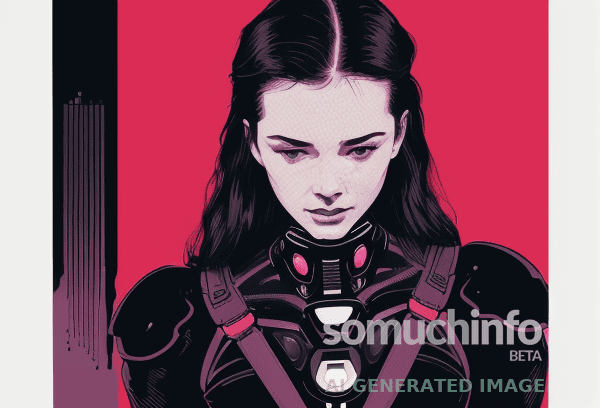
Framework 2: The ‘The AI Value Chain’
Another essential framework for balancing AI innovation and risk is the AI value chain. This involves identifying the key areas where AI can create value, such as process automation, decision support, and customer experience enhancement.
The ‘AI value chain’ consists of five stages:
The AI value chain refers to the series of activities and processes involved in developing, deploying, and maintaining 'artificial intelligence (AI) systems.'
It includes 'data collection,' annotation, model development, training, testing, deployment, and maintenance.
According to a report by MarketsandMarkets, the global AI market is expected to reach $190 billion by 2025, growing at a CAGR of 38%.
The value chain is divided into three main categories: data-driven AI, algorithmic AI, and hybrid AI.
-
Data Collection: Gathering relevant data to train and validate AI models.
-
Model Development: Designing and training AI models that meet business requirements.
-
Model Deployment: Integrating AI models into existing processes and systems.
-
Model Monitoring: Continuously monitoring the performance of AI models and making adjustments as needed.
-
Model Evolution: Regularly updating and refining AI models to ensure they remain relevant and effective.
By applying these two frameworks, organizations can develop a systematic approach to AI transformation that balances innovation with risk management. This enables companies to unlock the full potential of AI while minimizing its negative consequences.