Medical AI models face a critical challenge in understanding negation words, which can lead to inaccurate diagnoses and compromised patient care.
Medical artificial intelligence (AI) models face significant challenges when it comes to understanding negation words, such as ‘no’ and ‘not’. This limitation can have serious consequences for the accuracy and reliability of medical diagnoses.
Recognizing Negation: A Key Problem for Medical AI
Many AI models struggle to recognize negation words, which means they cannot easily distinguish between images labeled as showing a disease and those labeled as not showing it. For example, an X-ray image might be labeled as showing “signs of pneumonia” or “no signs of pneumonia”. If the AI model fails to recognize the negation word, it may incorrectly diagnose the patient with pneumonia.
Negation words are linguistic constructs used to negate or reject a statement.
In the context of artificial intelligence (AI), negation words play a crucial role in natural language processing (NLP) and machine learning algorithms.
They enable AI systems to understand and respond to negative statements, such as 'I don't like this' or 'This is not true.'
Common negation words used in AI include 'not', 'no', 'never', and 'none.' These words help AI systems to identify and process negations, which is essential for tasks like sentiment analysis and text classification.
The Implications of Negation Errors in Medical Imaging
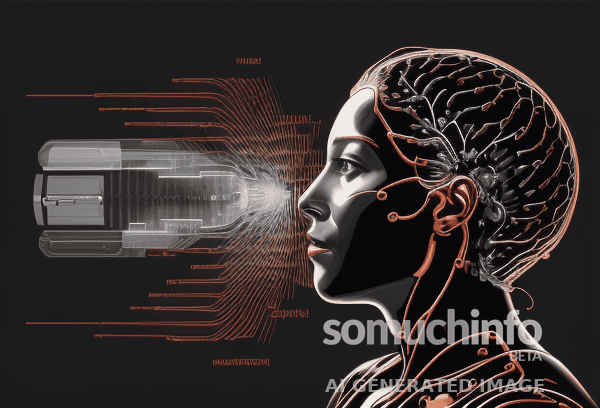
The inability of medical AI models to recognize negation words can have serious consequences for patient care. If physicians rely on these models for diagnoses, they may receive incorrect information, which could lead to delayed or inappropriate treatment. In some cases, this mistake could be catastrophic.
Artificial intelligence (AI) is increasingly being used to aid medical professionals in diagnosing diseases.
Machine learning algorithms can analyze large amounts of medical data, identifying patterns and anomalies that may indicate a specific condition.
Studies have shown that AI-assisted diagnosis can improve accuracy rates by up to 20%.
For example, Google's LYNA algorithm has been found to detect breast cancer with an accuracy rate of 99%.
As the field continues to evolve, AI is expected to play an increasingly important role in medical diagnosis and treatment.
Addressing the Challenge: New Directions in Natural Language Processing
Researchers are working to improve the ability of medical AI models to recognize negation words and other nuances of natural language. This requires advances in natural language processing (NLP) and machine learning algorithms that can better understand the context and intent behind human language. By addressing this challenge, we can improve the accuracy and reliability of medical diagnoses and enhance patient care.
Natural language processing has witnessed significant advancements in recent years.
Deep learning techniques, particularly neural networks and transformers, have improved the accuracy of text classification, sentiment analysis, and machine translation.
According to a report by Gartner, NLP adoption is expected to increase by 50% in the next two years.
Furthermore, the development of pre-trained language models such as BERT and RoBERTa has enabled faster training times and better performance on various NLP tasks.
- newscientist.com | AI doesnt know no – and thats a huge problem for medical bots