A novel benchmarking tool, IntersectionZoo, has been developed to evaluate the progress of deep reinforcement learning algorithms in solving eco-driving problems, providing insights into their generalizability and adaptability to various scenarios and environments.
New Tool Evaluates Progress in Reinforcement Learning for Eco-Driving
The Challenge of Eco-Driving in Urban Environments
Eco-driving, a technique used to reduce fuel consumption and emissions in urban environments, poses significant challenges for researchers. To develop effective solutions, it’s essential to address optimization problems involving many different agents, such as vehicles, and various factors influencing their emissions.
Eco-driving is a driving technique that aims to reduce fuel consumption and lower emissions.
It involves accelerating smoothly, maintaining a consistent speed, and anticipating traffic conditions.
By following these principles, drivers can save up to 15% on fuel costs and decrease their carbon footprint.
Additionally, eco-driving can improve road safety by reducing the number of accidents caused by aggressive driving habits.
Developing IntersectionZoo: A Benchmarking Tool for Eco-Driving
MIT researchers have developed a new benchmarking tool called IntersectionZoo, designed to evaluate the progress of deep reinforcement learning algorithms in solving eco-driving problems. This tool uses real-world traffic data to test the performance of algorithms and provide insights into their generalizability.
The Importance of Generalizability in Eco-Driving
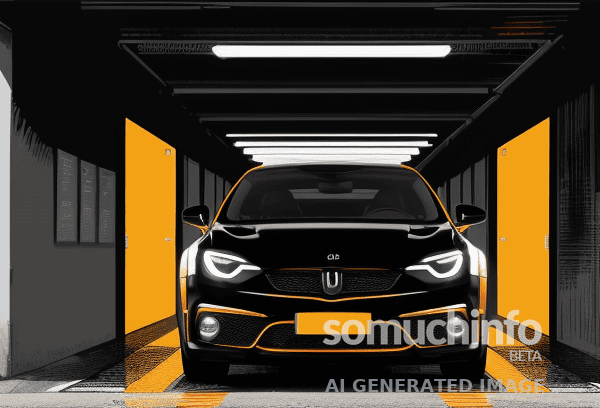
Generalizability is crucial for developing effective eco-driving solutions that can adapt to various scenarios and environments. IntersectionZoo addresses this challenge by providing a rich set of characteristics, including real-world data on traffic patterns, road conditions, and ‘vehicle types’.
Applying IntersectionZoo to Real-World Problems
The researchers aim to apply the newly developed benchmarking tool to address the impact of eco-driving in automated vehicles on emissions in cities. However, their primary goal is to support the development of general-purpose deep reinforcement learning algorithms that can be applied to various applications beyond autonomous driving.
Open Availability and Future Research Directions
IntersectionZoo and its documentation are freely available at GitHub, enabling researchers to access and build upon this valuable tool. As researchers continue to explore the capabilities of IntersectionZoo, they will also investigate new applications and extensions of eco-driving algorithms, including ‘video games, security problems, robotics, and warehousing’.
Conclusion
The development of IntersectionZoo represents a significant step forward in addressing optimization problems related to eco-driving in urban environments. By providing a standardized benchmarking tool, researchers can evaluate the progress of deep reinforcement learning algorithms and contribute to the development of more effective solutions for reducing emissions and improving air quality.