A groundbreaking new AI model, LinOSS, has been developed by researchers at MIT’s CSAIL, leveraging principles of forced harmonic oscillators to improve long sequence data handling and outperform existing state-of-the-art models.
Novel AI Model Inspired by Neural Dynamics from the Brain
Researchers at MIT’s Computer Science and Artificial Intelligence Laboratory (CSAIL) have developed a new artificial intelligence model inspired by neural oscillations in the brain. This model, called ‘linear oscillatory state-space models‘ (LinOSS), has been designed to improve how machine learning algorithms handle long sequences of data.
Artificial intelligence (AI) has been a subject of interest for decades, with its roots dating back to the Dartmouth Summer Research Project in 1956.
The field gained momentum in the 1980s with the introduction of expert systems and rule-based systems.
Today, AI is used in various applications, including virtual assistants, image recognition, and natural language processing.
According to a report by Grand View Research, the global AI market size is expected to reach $190.6 billion by 2025, growing at a CAGR of 38.1%.
The increasing adoption of AI in industries such as healthcare and finance has contributed to its rapid growth.
Leveraging Principles of Forced Harmonic Oscillators
The LinOSS model leverages principles of forced harmonic oscillators, a concept deeply rooted in physics and observed in biological neural networks. This approach provides stable, expressive, and computationally efficient predictions without overly restrictive conditions on the model parameters. By leveraging these principles, the researchers were able to create a model that can reliably learn long-range interactions, even in sequences spanning hundreds of thousands of data points or more.
Linear Oscillatory State-Space Models (LinOSS) are a class of mathematical models used to describe and analyze complex systems that exhibit oscillatory behavior.
These models are based on the concept of state-space representation, where the system's dynamics are described by a set of differential equations.
LinOSS models are particularly useful in fields such as signal processing, control theory, and systems biology.
They enable researchers to identify underlying patterns and relationships within complex data sets.
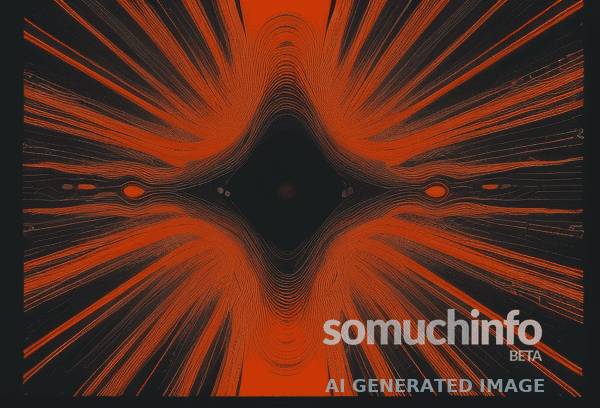
Significance and Performance
Empirical testing demonstrated that LinOSS consistently outperformed existing state-of-the-art models across various demanding sequence classification and forecasting tasks. Notably, LinOSS outperformed the widely-used ‘Mamba‘ model by nearly two times in tasks involving sequences of extreme length. The researchers’ work was recognized for its significance, with their research selected for an oral presentation at ICLR 2025 — an honor awarded to only the top 1 percent of submissions.
Machine learning algorithms are statistical models that enable computers to learn from data without being explicitly programmed.
These algorithms can be categorized into supervised, unsupervised, and reinforcement learning.
Supervised learning involves training on labeled datasets, while unsupervised learning involves identifying patterns in unlabeled data.
Reinforcement learning involves interacting with an environment to maximize a reward.
Popular machine learning algorithms include decision trees, random forests, neural networks, and support vector machines.
Potential Applications and Future Work
The team anticipates that the LinOSS model could significantly impact any fields that would benefit from accurate and efficient long-horizon forecasting and classification, including health-care analytics, climate science, autonomous driving, and financial forecasting. The researchers plan to apply their model to an even wider range of different data modalities and suggest that LinOSS could provide valuable insights into neuroscience, potentially deepening our understanding of the brain itself.
Their work was supported by the ‘Swiss National Science Foundation‘, the ‘Schmidt AI2050 program‘, and the ‘U.S. Department of the Air Force Artificial Intelligence Accelerator‘.