As AI systems increasingly navigate human environments, a recent study by Johns Hopkins University reveals the stark limitations of current technology in reading human behavior and understanding social dynamics.
A recent study by researchers at Johns Hopkins University reveals that artificial intelligence (AI) systems struggle to read the nuances of human behavior, a skill crucial for real-world applications like robotics and self-driving cars.
Artificial intelligence (AI) has undergone significant development since its inception in the mid-20th century.
The term was first coined by John McCarthy at a 1956 conference on AI.
Since then, AI has progressed from rule-based systems to machine learning and deep learning algorithms.
According to Gartner, the global AI market is expected to reach $190 billion by 2025, with applications in healthcare, finance, and transportation.
AI-powered chatbots are also increasingly used for customer service.
Self-driving cars, also known as autonomous vehicles (AVs), use a combination of sensors, GPS, and artificial intelligence to navigate roads without human input.
The technology has been in development since the 1980s, with early experiments focusing on sensor-based systems.
Modern AVs rely on sophisticated software algorithms and machine learning to recognize patterns and make decisions in real-time.
According to a report by Grand View Research, the global autonomous vehicle market is expected to reach $7.1 trillion by 2025.
While AI excels at solving complex logical problems, it falls short when understanding social dynamics. This is a critical issue, as human drivers make decisions based on not just traffic signals but also predicting how other drivers will behave. To test AI’s ability to navigate human environments, researchers designed an experiment in which both humans and AI models watched short, three-second videos of groups of people interacting at varying levels of intensity.
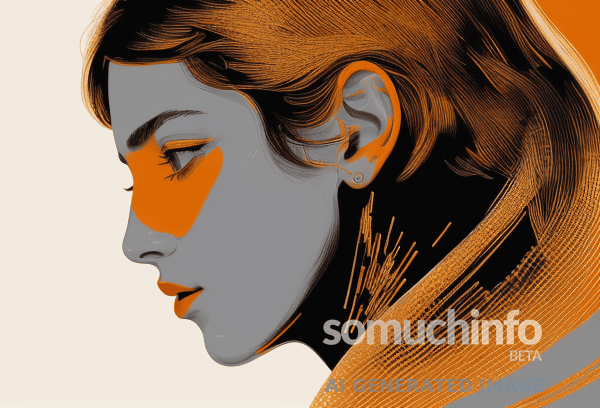
The experiment revealed a stark difference between human and machine performance. Among the 150 human participants, evaluations of the videos were remarkably consistent. In contrast, the 380 AI models’ assessments were scattered and inconsistent, regardless of their sophistication. This finding highlights key limitations of current AI technology, particularly when it comes to predicting and understanding how dynamic systems change over time.
Understanding the thinking and emotions of an interaction involving multiple people can be challenging even for humans. ‘Konrad Kording‘, a bioengineering and neuroscience professor at the University of Pennsylvania, noted that ‘There are many things we might be better at’ when it comes to social interactions. ‘Dan Malinsky‘, a professor of biostatistics at Columbia University, emphasized that the study highlights the need for AI systems to understand the nuances of human behavior.
Researchers believe the problem may be rooted in the infrastructure of AI systems. AI neural networks are modeled after the part of the human brain that processes static images, which is different from the area of the brain that processes dynamic social scenes. ‘Kathy Garcia‘, a co-author of the study, stated that ‘It’s not enough to just see an image and recognize objects and faces. We need A.I. to understand the story that is unfolding in a scene.‘
The findings of this study have significant implications for real-world technologies like self-driving cars. As AI systems are increasingly used in these applications, it is essential to address the limitations in their ability to understand human social dynamics. By acknowledging these challenges and working towards developing more sophisticated AI models, we can create safer and more reliable technologies that benefit society as a whole.