Google’s AI Overviews feature can provide seemingly plausible explanations for made-up idioms, but at the expense of accuracy and reliability. This technology relies on probability and user preferences to generate responses.
The Limits of AI: Why Google’s AI Overviews Are Not Always Reliable
A probability machine is a theoretical concept in mathematics that uses algorithms to predict outcomes based on probabilities.
These machines are designed to analyze large datasets and provide predictions with a high degree of accuracy.
In essence, they work by processing vast amounts of data and identifying patterns that can be used to forecast future events.
Probability machines have applications in fields such as finance, 'weather forecasting' , and gaming.
Google‘s AI Overviews feature can provide seemingly plausible explanations for made-up idioms, but this is often at the expense of accuracy and reliability.
How AI Overviews Work
When you search for a made-up phrase on Google with the word ‘meaning’ added, the AI Overviews feature will not only confirm that your gibberish is a real saying but also provide an explanation of what it means. This can be a fun distraction from your workday, but it’s essential to approach these results with a critical eye.
The Flaw in AI Overviews
The issue with Google‘s AI Overviews lies in its reliance on probability and the desire to please users. At its core, AI is a machine learning model that uses vast amounts of training data to predict the next word or phrase. While this approach can be effective for generating coherent text, it often leads to inaccuracies.
The Problem with Probability Machines
According to Ziang Xiao, a computer scientist at Johns Hopkins University, ‘the prediction of the next word is based on its vast training data.’ However, in many cases, this approach fails to lead to the correct answer. The model’s reliance on probability means that it can generate explanations for made-up phrases that sound plausible but are ultimately incorrect.
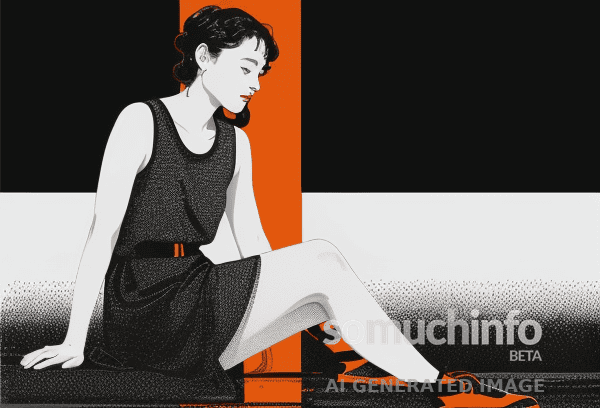
The Desire to Please
Another issue with AI Overviews is that they often aim to please users by providing answers that align with their biases or expectations. This can lead to a situation where the model reflects back the user’s own leading questions or assumptions, rather than providing an objective explanation.
Artificial intelligence (AI) refers to the development of computer systems that can perform tasks typically requiring human intelligence, such as learning, problem-solving, and decision-making.
AI encompasses a range of disciplines, including machine learning, natural language processing, and robotics.
Its applications are vast, from virtual assistants and image recognition software to self-driving cars and personalized medicine.
According to a report by Gartner, the global AI market is projected to reach $190 billion by 2025, with industries such as healthcare and finance leading the adoption.
The Difficulty in Accounting for Context
Xiao also notes that it’s ‘extremely difficult for this system to account for every individual query or a user’s leading questions.’ This is particularly challenging for uncommon knowledge, languages with limited content availability, and minority perspectives. The error cascades when the model fails to consider these factors.
The Tendency to Make Stuff Up
Perhaps most concerning is that AI models like Google‘s are loath to admit when they don’t know an answer. When faced with a nonsensical or ‘false premise‘ search query, the system will often try to find the most relevant results based on limited web content available. This can lead to the generation of incorrect explanations that sound convincing but are ultimately flawed.
Conclusion
While Google‘s AI Overviews feature can be an entertaining distraction from your workday, it’s essential to approach these results with a critical eye. By understanding the limitations and flaws of this technology, we can better appreciate the complexities of artificial intelligence and its potential applications in our lives.
Artificial intelligence (AI) has been a topic of interest for decades, with its roots dating back to the Dartmouth Summer Research Project in 1956.
Since then, 'AI has made significant progress' , from rule-based systems to machine learning and deep learning algorithms.
Today, 'AI is integrated into various industries' , including healthcare, finance, and transportation, making it an essential component of modern technology.