As medical artificial intelligence tools gain traction in healthcare, concerns arise over inadequate testing protocols, with some experts questioning their efficacy and accuracy.
Artificial intelligence algorithms are being increasingly integrated into various aspects of healthcare, from breast cancer screenings to clinical note-taking. While companies claim that these tools will make medicine more efficient and reduce the burden on doctors and other healthcare workers, some experts question whether they work as well as claimed.
Medical AI tools are transforming the healthcare industry by improving diagnosis accuracy, streamlining clinical workflows, and enhancing patient outcomes.
These tools utilize machine learning algorithms to analyze vast amounts of medical data, identify patterns, and provide insights that human clinicians may miss.
According to a study, AI-assisted diagnoses have shown an average accuracy rate of 87%, compared to 63% for human clinicians alone.
By automating routine tasks and freeing up clinical staff for more complex cases, medical AI tools are revolutionizing healthcare delivery.
The current benchmark tests used to evaluate medical AI models are based on evaluations that use medical student exams, such as the MCAT. However, a review of studies evaluating health care AI models found that only 5 percent used real patient data. Moreover, most studies evaluated large language models (LLMs) by asking questions about medical knowledge, rather than assessing their ability to perform tasks such as writing prescriptions or having conversations with patients.
The Problem with Current Benchmark Tests
Computer scientist Deborah Raji and her colleagues argue that the current benchmarks are distracting and do not accurately measure actual clinical ability. They also fail to account for the complexities of real-world cases that require nuanced decision-making. Furthermore, these tests are based on physicians’ knowledge and do not properly represent information from nurses or other medical staff.
Deborah Raji is a researcher and advocate for fairness in artificial intelligence.
She focuses on the intersection of AI, ethics, and bias, particularly in image classification models.
Her work aims to improve the accuracy and fairness of AI systems.
Raji has published research papers on AI bias and has spoken at conferences about the importance of diversity and inclusion in AI development.
Raji explains that the whole field should not obsess over current benchmarks, which are often geared towards question-answering and knowledge recall. Instead, she advocates for developing evaluations of how LLMs perform when responding to complex and diverse clinical tasks.
Large Language Model (LLM) performance is evaluated using metrics such as perplexity, accuracy, and F1 score.
Perplexity measures a model's ability to predict the next word in a sequence, while accuracy assesses its overall correctness.
F1 score combines precision and recall for multi-class classification tasks.
Benchmarks like GLUE and SuperGLUE provide standardized evaluations for LLMs.
These metrics and benchmarks help developers and researchers compare and improve their models' performance.
Creating Better Evaluations
Raji suggests several strategies for creating better evaluations, including:
- Interviewing domain experts to understand the actual practical workflow in healthcare.
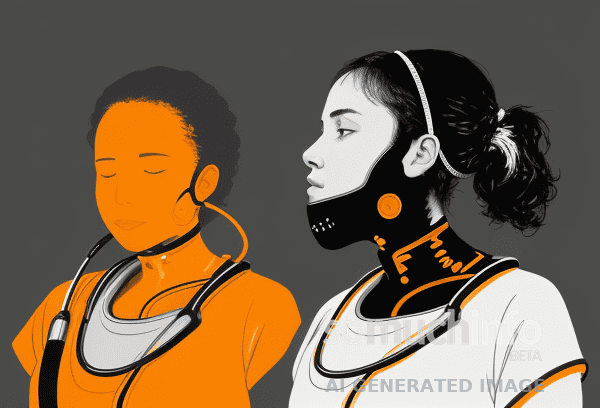
-
Collecting naturalistic datasets of pilot interactions with the model to see the types of queries that people put in and the different outputs.
-
Using ‘red teaming,’ which involves actively gathering a group of people to adversarially prompt the model.
-
Gathering information from actual hospitals about usage data, workflows, and anonymized patient information.
The Need for Specialized Benchmark Testing
Raji emphasizes the importance of nuance in task design when creating benchmark tests. For example, a benchmark geared towards question-answering is very different from one that validates the model on summarizing doctors’ notes or doing questioning and answering on uploaded data.
Policies and Frameworks Needed to Create Better Evaluations
Raji calls for researchers to invest more in thinking through and constructing evaluations that are more grounded in reality. She also suggests that institutions, such as hospitals, should share information about the workflows that they integrate AI systems into, which can help create better evaluations.
At the vendor level, sharing information about current evaluation practices is also essential. This will help identify the gap between what vendors currently do and what could be more realistic or grounded in reality.
Advice for Those Working with Medical AI Models
Raji advises people working with these models to be more thoughtful about the evaluations they focus on. She encourages them to pay attention to constructing valid representations of what they hope the models do and their expectations for these models once they are deployed.
In conclusion, while medical AI tools show promise in improving healthcare efficiency and reducing the burden on doctors and other healthcare workers, it is essential to address the flaws in current testing methods. By creating more realistic and nuanced evaluations, we can ensure that these tools live up to their potential and provide better care for patients.
- sciencenews.org | Medical AI tools are growing, but are they being tested properly?